
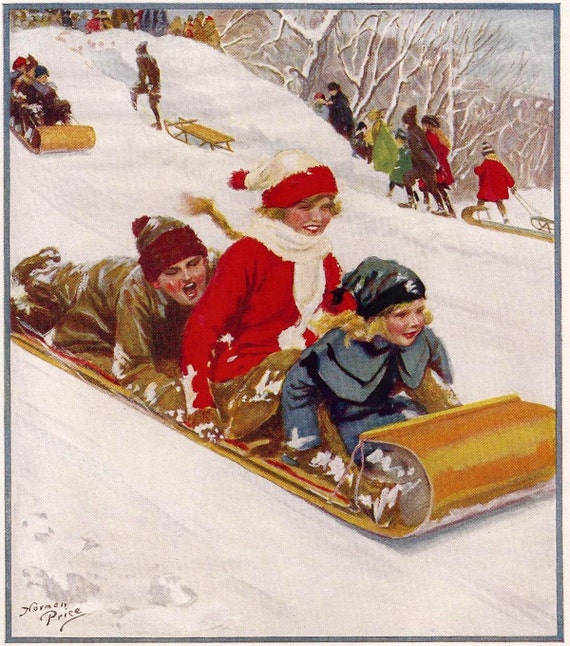
As a result, the proposed method, modified SSR-NET (MSSR-NET), outperforms the baseline SSR-NET and other state-of-the-art methods quantitatively and qualitatively. To improve the spatio-spectral feature extraction ability of this baseline method and at the same time to keep the network shallow, we add long and short skip connections and two convolutional blocks. et al., 2021) architecture, which is a state-of-the-art HSI SR network. In this paper, a novel two-input fusion HSI SR method is proposed as a modification of the baseline method, SSR-NET ( Zhang X. There are two special cases of hyperspectral image super-resolution when only one of the inputs (HR MSI or LR HSI) is given. Therefore, the most practical way to obtain higher resolution imaging for both spatial and spectral domains is to fuse these two types of inputs, HR MSI and LR HSI, by taking advantage of spatial information of the first input, and a correlation among the spectral bands of the second input. Instead, multispectral cameras capture multispectral images (MSI) with much higher spatial resolution than HSI cameras. Unfortunately, hyperspectral imaging systems mainly focus on captioning higher spectral resolution because of the hardware limitations, which adversely affect spatial resolution. Moreover, for extracting more information, some methods apply HSI super-resolution (SR) as a preprocessing step for other computer vision tasks, such as dehazing ( Makarau et al., 2014 Gan et al., 2016 Mehta et al., 2020, 2021) and object detection ( Pham et al., 2019 Yan et al., 2021).

Hyperspectral imaging is widely used in areas such as anti-spoofing ( Kaichi and Ozasa, 2021), food quality and safety assessment ( Feng and Sun, 2012), medical diagnosis ( Fei, 2020), precision agriculture ( Rascher et al., 2007). Due to the significant increase of information that hyperspectral images (HSI) provide with respect to RGB images, they are considered beneficial for many computer vision tasks, especially in cases when three channels of RGB images are not enough to identify and distinguish objects and materials ( Segl et al., 2003 Khan et al., 2018 Cavalli, 2021). Unlike conventional cameras capturing images with three spectral bands (RGB), hyperspectral imaging systems capture hundreds of spectral bands of different wavelengths.
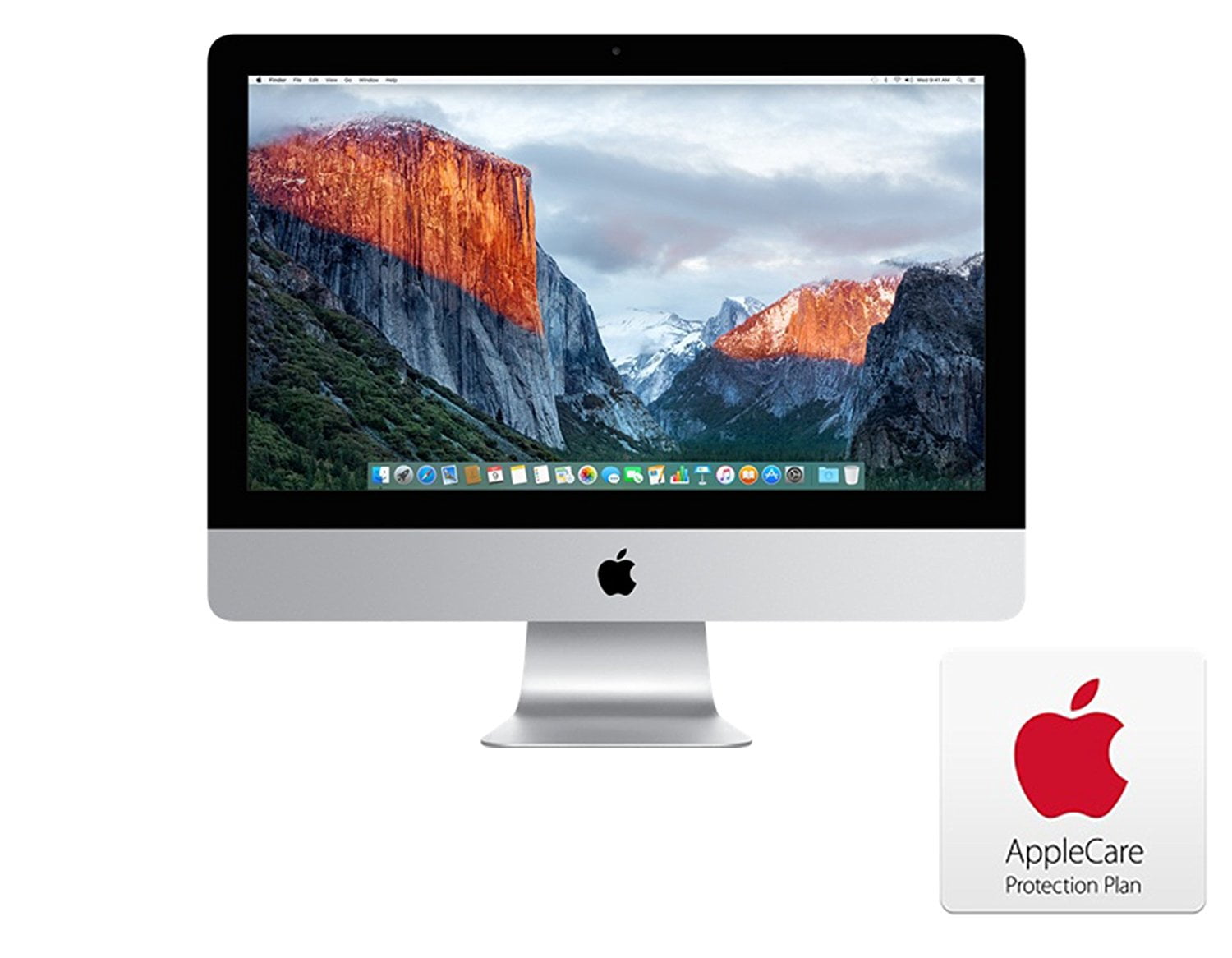
The hyperspectral image super-resolution is a fast-growing research area in computer vision, particularly due to technical difficulties of high-resolution hyperspectral data acquisition with both high spatial and spectral resolutions. It is also shown that, in some cases, our method with a single input, HR MSI, can provide a comparable result with that achieved with two inputs, HR MSI and LR HSI. Specifically, for the fusion setup with two inputs, obtained by 32× spatial downsampling for the low-resolution hyperspectral (LR HSI) input and 25× spectral downsampling for high-resolution multispectral (HR MSI) input, a significant improvement of the quality of super-resolved HR HSI over 4 dB is demonstrated as compared with SSR-NET. Numerical experiments show both the visual and quantitative superiority of our method. The feature extraction ability is improved compared to SSR-NET and other state-of-the-art methods, while the proposed network is also shallow. Computational Imaging Group, Faculty of Information Technology and Communication Sciences, Tampere University, Tampere, FinlandĪ fast and shallow convolutional neural network is proposed for hyperspectral image super-resolution inspired by Spatial-Spectral Reconstruction Network (SSR-NET).

Shushik Avagyan*, Vladimir Katkovnik and Karen Egiazarian
